Our planet is in the midst of its sixth mass extinction, the worst since dinosaurs roamed the earth, 65 million years ago. Every 14 minutes, an elephant is killed in Africa, while a Rhino is slaughtered every 11 hours. It is because of countless direct and indirect human actions, such as poaching, overpopulation, habitat destruction and climate change, that our with data science company planet’s rich biodiversity is taking such a huge hit. By Ganes Kesari, co-founder and head of analytics of data science company Gramener
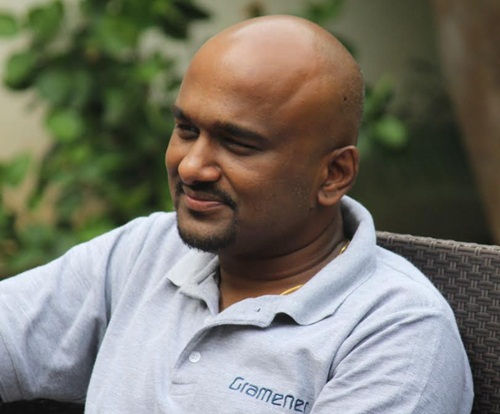
Our planet is in the midst of its sixth mass
extinction, the worst since dinosaurs roamed the earth, 65 million years ago. Every 14 minutes, an elephant is
killed in Africa, while a Rhino is slaughtered every 11 hours. Over 14,000 species in the US are listed as
endangered, many of which have been around for millions of years. The responsibility for this crisis lies with us, humans. It is because of countless direct and indirect human actions, such as poaching, overpopulation, habitat destruction and climate change, that our planet’s rich biodiversity is taking such a huge hit.
“We know that species are becoming extinct at a rapid pace – at least 1,000 times more quickly than would be expected in the absence of human activities.” -
Lucas Joppa, Chief Environmental Officer at Microsoft.
Technology: friend or foe?
Environmentalists are facing insurmountable odds as poachers resort to the latest technology in night-vision goggles, military grade weaponry, and sophisticated transportation. Thankfully, it’s the state-of-art technology that’s also giving a boost to conservation efforts around the world.
The revolutionary advances in Artificial Intelligence (AI) have unlocked the ability to rapidly process a variety of signals, identify risks from them accurately, and provide real-time alerts to the conservationists. In this fight against human greed, AI models have turned into an unlikely ally, helping save our biodiversity, paradoxically from our own hands.
The immense power of Deep Learning models
Deep Learning models, a type of AI system, are particularly effective at pattern recognition and identification. For example, when these models are given thousands of pictures of whale sharks, they can
learn to spot a unique whale shark from a future sighting, with remarkable accuracy. A feat that humans would struggle to match.
In addition, these models are particularly
effective at doing this with unstructured data such as images, videos and audio clips -- formats that computers have been struggling with for decades. The deep learning models are first taught with pre-labelled images, and then learn their lessons by running hundreds of iterations on high-performance, GPU infrastructure. These trained models can then instantly identify any new picture that they’re presented with.
AI for Social Good
The
Nisqually Foundation in the US recently faced the challenge of identifying salmon species from underwater camera trap videos, a task which required the precious time of its expert biologists.
Microsoft AI for Earth and
Gramener devised a
deep learning solution that was able to process hundreds of hours of video to identify frames with the snapshots of fish. The application was then able to identify the salmon species with high accuracy, saving over 80% of human effort in the processing and identification of salmon from the videos.
Such AI solutions hold immense potential in identifying hard-to-track species, provided there are enough labelled images available to train the models. Adding to the efforts are citizen environmental groups such as
iNaturalist, which act as thriving online social networks that exist with the primary aim of mapping and sharing observations of biodiversity across the globe.
Leveraging the rich database of millions of crowdsourced pictures of plants and animals, Microsoft and Gramener built an AI solution that can name them real-time from over 5,000 species. This solution has been packaged as a
public API on the Microsoft AI platform and is available for use by citizen scientists and NGOs.
Penguin Watch is a citizen science project that captures time-lapse pictures of penguin colonies using fixed cameras in several locations in Antarctica. However, if the the identification and classification of species is labor-intensive for humans, spotting changes in their population counts across years presents an even bigger challenge, especially as these multi-year pictures run into the hundreds of thousands.
AI has come to the rescue of these penguins, who are threatened by climate change and human disturbances. Density-based deep learning algorithms learn from the crowd-sourced annotated images, wherein volunteers place dots to identify every penguin. Armed with this training, the models built by researchers at the
University of Oxford and machine learning teams around the world provide accurate counts of the birds in each picture. This intelligence helps researchers better understand threats to the ecosystem and answer deeper questions on wildlife dynamics.
Wildbook is an open source platform from
Wildme that uses photographs of skin patterning behind the gills of each shark to uniquely identify Whale Sharks from public photographs submitted from the ocean sightings. This makes it possible for members of the public to contribute to the conservation of this beautiful species, just by submitting their holiday snaps.
Over on the African continent, national parks pose a different kind of conservation challenge for elephants. With tens of thousand square miles of roadless territory and a very dispersed elephant population, monitoring the elephant population is tough and poaching has seen an unprecedented rise. AI models can be put to use here in conjunction with aerial defence efforts by organizations such as
Save the Elephants, wherein anti-poaching aircrafts capture continuous shots of aerial pictures of the landscape. AI models can then spot elephant herds from thousands of such pictures and accurately tell them apart from images of cattle or other wildlife to identify concentrations of vulnerable elephants.
With all this in mind, there is a glimmer of hope that these efforts to conserve our planet’s biodiversity will be worth it. Streaming data feeds generated by the latest smart devices can be converted into actionable intelligence by AI. With an ability to
uniquely identify, name and
monitor all of our endangered species, AI models will soon be able to
alert us of the next poaching incident, enabling rangers to reach the spot, even before the poachers.
Snapshot of Salmon species detection solution developed for Nisqually Foundation, by Gramener and Microsoft
Species Classification API built by Gramener and Microsoft
AI Detection solution built by Gramener and Microsoft that works on Aerial images captured by ‘Save the Elephants’ foundation (aerial shot on the left; AI detection on the right)
The author is a co-founder and head of analytics at Gramener, and helps NGOs adopt AI models in their conservation efforts. He is an Industry recognized speaker in Data science and is recognized as a top writer in 'Artificial Intelligence' on Medium.